The Chip Insider® - Al: Hot or Hype?
June 2, 2023
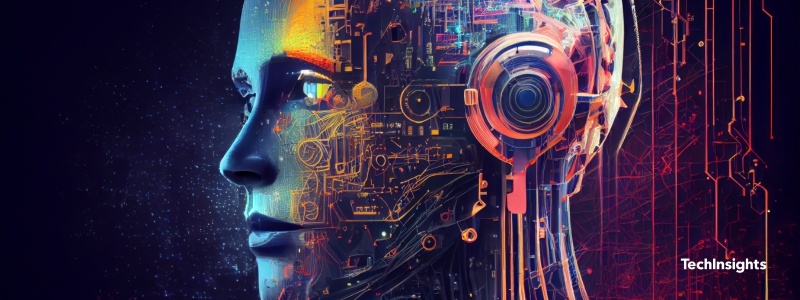
Summary:
Al: Hot or Hype? Last week, the stock market went crazy over anything associated with AI, while the rest of the market languished. Nvidia blew up the market with Jensen’s amazing results and outlook. Following it, Marvell blew out the market with results and by mentioning the term ‘AI’ 97 times in its call… which contrasts to hardly a mention a year ago. However, Marvell’s claim to ... It’s selling blue jeans next to Nvidia’s picks and shovels needed to mine the gold in AI…
While we should certainly congratulate the financial relations people who figured out the relationship between the term ‘AI’ and stock prices in an otherwise gloomy market, the real question is what’s different this time? After all, Jensen Huang and Gary Dickerson have been talking up AI driving growth in the semiconductor supply chain for years. Is this just hyped-up investors who can’t think of anywhere else to put their money? Or has demand for AI suddenly turned hot? There is reason to believe it is the latter. If it is hot, how does one articulate it to the world in a way that doesn’t sound like you’ve jumped on the hype cycle and are just riding it around in circles?
The opportunity for Nvidia has been to attack the scale issues of AI with Gary Dickerson’s PPACt strategy which brought more Performance, with less Power, in a smaller Area, with lower Cost, in a predictable time cadence. At a silicon level, AI will … The reason comes back to … Profit = f (TVOO + PPACt).
But it doesn’t stop here. The reason why Nvidia developed its first CPU, Grace (note the homage to the fabled computer scientist Grace Hopper) is to support Hopper, its GPUs. GPU’s superior ability to do … But transformer... My guess is Nvidia saw this need emerging and has tailored Grace to work with Hopper in ways …
Maxims: There are two social competitive strategies: Network and Ecosystem
“No computer is ever going to ask a new, reasonable question.
It takes trained people to do that.” – Grace Hopper
Al: Hot or Hype?
Last week, the stock market went crazy over anything associated with AI, while the rest of the market languished. Nvidia blew up the market with Jensen’s amazing results and outlook. Following it, Marvell blew out the market with results and by mentioning the term ‘AI’ 97 times in its call… which contrasts to hardly a mention a year ago. However, Marvell’s claim to being a part of the expected AI boom does not rest on having market advantage in the processors essential to making AI work. Instead, its expertise is in networking chips, which are only complementary. It’s selling blue jeans next to Nvidia’s picks and shovels needed to mine the gold in AI. While Marvell wasn’t close to Nvidia’s 247% gain since the bottom last October, it did jump from up 23% over this timeframe two weeks ago to close up 77% the day after its conference call last Friday.
While we should certainly congratulate the financial relations people who figured out the relationship between the term ‘AI’ and stock prices in an otherwise gloomy market, the real question is what’s different this time? After all, Jensen Huang and Gary Dickerson have been talking up AI driving growth in the semiconductor supply chain for years. Is this just hyped-up investors who can’t think of anywhere else to put their money? Or has demand for AI suddenly turned hot? There is reason to believe it is the latter. If it is hot, how does one articulate it to the world in a way that doesn’t sound like you’ve jumped on the hype cycle and are just riding it around in circles?
AI has been around for a long time. It was first hyped more than fifty years ago when leading universities sold the military on the idea of using computers for image recognition with dreams of self-guided, terrain-hugging cruise missiles. It died down until almost exactly 26 years ago when – on May 11, 1997 – IBM’s Deep Blue computer beat world chess champion Garry Kasparov. The hype then quieted down until 2011, when IBM’s Watson beat Jeopardy champion Ken Jennings. Five years later, Google’s AlphaGo beat Go champion Lee Sedol in 2016. And then it died down only to come back soon after OpenAI released ChatGPT in November of 2022, which democratized AI in a way similar to the release of the Personal Computer with word processing and spreadsheet programs in the eighties.
The reason why AI continued to attract investment over the decades was largely due to its success with taking humans out and putting far more accurate machines in harm’s way. The reason why AI continued to attract media hype over the decades was its ability to outplay the best humans at games. The reason why AI is attracting hype in the investment community today is its potential to be monetized in a way unseen since Apple’s release of the iPhone at the hardware level and Google’s release of the automated search engine at the software level.
But why now? There is a co-dependency between the effect of Moore’s Law on compute power and the development of AI algorithms. In the sixties, AI was a simple merger of computers and statistics that relied on linear regression or time-series methods with math no more complex than 2D matrix inversions. While there were attempts at 3D matrix inversion in the seventies that yielded interesting results – such as pegging the inflation problems of the day on monetary expansion in the sixties to fund the Vietnam War and Great Society – they required supercomputers. Few could afford them. The impact of Moore’s Law on driving down cost while driving up compute Performance-per-Watt solved this exponentially over time. It also enabled the invention of the FMRI, which allowed researchers to map the brain’s neural network. With this came improved AI methods such as Convolutional and Recurrent Neural Networks (CNNs and RNNs). The latest breakthrough that explains the ‘WHY NOW’ question is the development of transformers and foundational models.
Transformers solve the sequence transduction problem of CNNs and RNNs, which need to be trained by a human (and is a reason why attempts at self-driving cars failed). Sequence transduction is simply the process of transforming an input into an output. For example, the input might be a new picture of a friend taken with your phone that it can identify as Gordon because you have other pictures of Gordon on your phone that you have labeled as being of Gordon. But if you’ve never done this, your phone can’t identify the person in the model. Transformers don’t need data labeled by humans. They train themselves by taking massive amounts of data and looking for correlations. It’s pretty similar to what a human does. So for example, a transformer might match the time the photo was taken to your calendar, which showed you meeting Gordon and guess the picture was of Gordon. Because transformers with large data sets give them LSTM (Long-Short Term Memory) they can improve the accuracy of guess by looking for other matches of the image to name. What it chooses to look for is called an attention model and gives a transformer the ability to train itself, which is called unsupervised learning. It is also what is meant by the term Generative AI, which means the transformer can generate new content that didn’t exist before.
Expanded out to data sets sized as large as 1021, transformers become what the Stanford Institute for Human-Centered Artificial Intelligence named Foundational Models. The problem is that it puts us back in the scale issues of the sixties, because Foundational Models can cost hundreds-of-millions of dollars and use many kWhs to develop. Foundation models can be built around many domains, such as code, legal, physics, chemistry, imaging, and the most hyped: Large Language Models. Once the model is built, it can be tuned, which is different from the training used in CNNs and RNNs. Tuning is often about matching word meaning to the domain you’re in. For example, this content could be tuned so that the term ‘cycle’ is understood to be repeating series of events and not a bicycle. The phrase from above, “jumped on the hype cycle and are just riding it around in circles,” is very confusing to AI systems since I’ve interchanged both definitions to get a smile out of the reader with the imagery it brings to mind. It's also why AI systems struggle with jokes. A well-tuned foundational model, anchored with the knowledge the cycles in this content are about business, would not make the mistake that the article is about bicycles.
Tuning is also what addresses the trust issue with foundational models. Letting a transformer loose in the internet is like letting your toddler loose on a high school playground. The language your toddler returns with after a few days of playing there will definitely be unacceptable. Foundational models are less likely to filter out hate speech on the internet than a high school kid is. That’s why if you plan to use them in your business, they need lots of tuning.
Enter Large Language Models: ChatGPT is an LLM and has generated plenty of controversy, which I won’t go into here. As for monetizing LLMs, there are plenty of opportunities that don’t require spend levels above $100M, as long as someone else has paid the fixed cost of developing the LLM. An LLM can be tuned for specific inference purposes with less than a million. This is how the business model will evolve. Currently, it’s running on the old URL model: Ubiquity first, Revenues Later. Microsoft, which bought ChatGPT is putting it into its Office™ suite of products.
The opportunity for Nvidia has been to attack the scale issues of AI with Gary Dickerson’s PPACt strategy which brought more Performance, with less Power, in a smaller Area, with lower Cost, in a predictable time cadence. At a silicon level, AI will keep demand going. Flops-per-Watt will be critical, since a ChatGPT search uses 10-to-100X the electricity of a typical search. Nvidia’s Hopper is one of the most expensive chips in the world, because it has 83B transistors. With over 1500 process steps, chips like this have something like 100 miles of copper wiring. Yet companies are lined up to buy it. The reason comes back to Total Value of Ownership (Profit = f (TVOO + PPACt).
But it doesn’t stop here. The reason why Nvidia developed its first CPU, Grace (note the homage to the fabled computer scientist Grace Hopper) is to support Hopper. GPU’s superior ability to do matrix inversion is why it’s so good at doing probabilistic math needed for neural networks. But transformer math is deterministic, for which CPUs are better. My guess is Nvidia saw this need emerging and has tailored Grace to work with Hopper in ways optimized for foundational models.
FYI: I’ve underlined the key words to add your AI vocabulary.
Maxims: There are two social competitive strategies: Network and Ecosystem
Microsoft and the PC made the concept of a Network advantage popular. A network advantage follows Metcalfe’s Law, which states that the value of any network is proportional to the square of the number of connected users. Apple made the concept of an Ecosystem advantage popular with the 1-2 punch of iTunes and the iPod. Think of ecosystem advantage as an upside-down network advantage. Instead of users connecting to a single network, an ecosystem’s value is proportional to the square of the number of supply vectors connected both vertically and horizontally.
Whenever a high-value network emerges, a strong ecosystem will emerge to supply it. So people often think of the WinTel PC alliance as the critical market determinant, but in reality it was the subsequent emergence of a broad-based ecosystem of competing suppliers. Proof is that shocks to the WinTel alliance like Linux and AMD did not weaken the PC platform, they strengthened it.
Apple’s dominance with the iPad is not just design and marketing. They combined both a strong network and ecosystem advantage when they first hit the market with it. The network arose out of the iPhone, which Apple consciously decided to introduce first, even though the iPad’s development predated it. The iPhone gave them the user base and a network via AT&T, etc. The App Store brought an army of application developers right out of the box. Meanwhile, the brilliant orchestration of their supply base brought it off without a hitch.
You succeed with a closed ecosystem if you are large enough and have a dedicated base of users. Nikon’s success in cameras is a good example. Their ecosystem is the many lenses and accessories that make applications for their SLRs pretty limitless. Applied Materials did the same with its service and support organization. Nvidia’s success, like Apple’s has been to pair its hardware with software that makes it easy to do AI with their products in their walled garden.
For more, search the ‘Maxims of Tech’ category in the learn section of the Chip History Center.
G. Dan Hutcheson
Manufacturing Analysis
Dan is Vice Chair of TechInsights Inc. He is a recognized authority on the semiconductor industry, winning SEMI’s Sales and Marketing Excellence Award in 2012 for “empowering executives with tremendous strategic and tactical marketing value" through his e-letter, The Chip Insider®; his book Maxims of Hi-Tech, and his many interviews of executives.
As some industry leaders have said, “He is the marketing voice and expert for the industry.” “Dan has methodically captured the essence of the industry and produced it in such a way for all to benefit … He has been such an integral part of the industry for so long, it is difficult to imagine the industry without his contributions.”
Dan’s public work on the industry has often focused on challenging predictions of the demise of Moore’s Law that date back decades by demonstrating how doomsayers have been outpaced by emergent behavior through the innate ability of technologists to innovate.